UX Design Internship
In summer 2023, I joined Spaero Bio, a startup aimed at automating biological experiments across life sciences. I was lucky enough to work across several design roles, identifying and solving user needs from the initial user research through to feature prototyping for v 1.0 of the product.


During my 3 months at the company, the rest of the team was working on getting the MVP of the product shipped to customers. I was more of an independent contributor working on version 1.0 of the product.
My job was to study lab automation in academia and build design concepts to be included in the next iteration of the product.
My role


Healthcare and pharmaceuticals have undergone a profound transformation over the years, shifting from traditional approaches to highly advanced and technology-driven systems.
Lab automation has scaled up experiments while allowing scientists to focus on high-level science
But...
the technology has outpaced its adoption and ease of use.

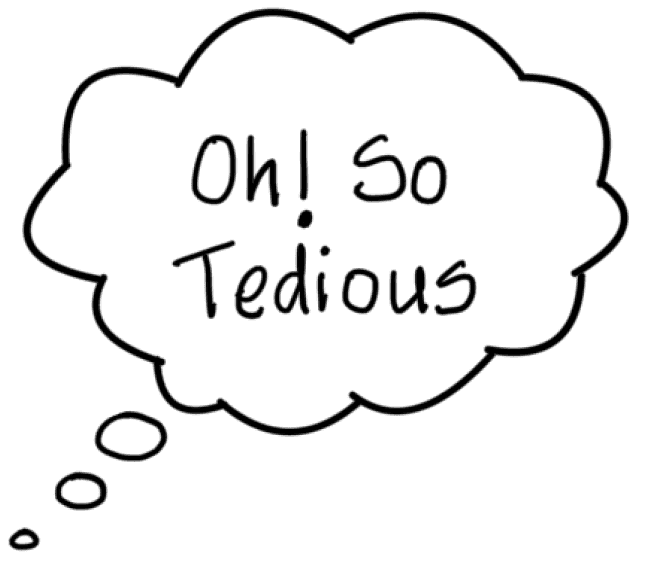
Traditional Life Sciences

Modern Life Sciences

The biggest advantages of lab automation

Walk Away Time
Automation ensures better use of time for scientists to focus on high-level scientific problems rather than low-level tasks like pipetting.
Efficiency and Speed
Lab automation can perform repetitive tasks much faster and with higher precision than humans.
Accuracy and Reproducibility
The accuracy that lab automation provides is critical to prevent misleading results and wasted resources.
Data Monitoring and Quality
High-throughput experiments generate large volumes of data. Automation helps handle, store and analyse data.
OEM softwares are powerful but difficult to learn leading to a slight inertia towards adoption
Steep learning curve
OEM softwares are difficult to learn
Slight inertia towards adoption
This makes scientists and first-time users more reluctant towards adopting automation
Not beginner friendly
They are not intuitive enough for first-time users to adopt
Scientists manually run experiments
Scientists prefer to do things by hand which is inefficient and prone to errors
Gaps in knowledge transfer
Due to differing expertise between scientists and automation engineers, experiments are more error prone due to gaps in knowledge transfer
Requires and Automation Engineer
For high-throughput experiments, automation is a must and needs an additional automation engineer

So what did I do to make lab automation easier?

The primary user is a bench scientist trained in biological or chemical sciences. They work in the pharmacology and drug discovery fields. They have traditionally been designing and performing experiments by hand. This, while accurate and quick for smaller experiments, is not feasible for drug discovery or cell culture that requires thousands of samples.
Understanding the user
After I developed sufficient knowledge of the industry and problem space, my focus shifted to more primary research. Being in a college town, I was able to interview and observe scientists at labs in my own university.
User Needs

How Might We
improve scientist’s experience of creating experiments in lab automation setups by making it more intuitive and transparent?
Competitive Analysis
What did I learn from competitive analysis?
Most competitor products had different areas of focus and approached experiment creation differently. But, they seemed to have a few common drawbacks:
Why do a competitive analysis?
To help understand the current state of the market, what worked and what was lacking
Choice of competitors
Based on brand popularity
Analysis lenses
Ease of use, barrier to entry, transparency of system status
Complicated labware definition
The experience of defining starting liquids, their volumes, concentrations and labware included in the experiment were confusing
Unintuitive experiment creation
Creating an experiment did not make much logical sense and did not follow the mental model of the scientist
Unintuitive simulations
Simulations do not convey much information that is important to the experiment such as volumes and concentrations moved
User Journey
While observing my target scientists, I noted typical steps taken in order to go from the high-level science of their experiment to the process of transcribing it to instructions for the liquid handler robot. The main steps involved are:

Bench Science
The high-level science performed by the scientist in a laboratory setting. This includes hands-on experiments using various laboratory equipment.
Experiment Creation
The first step of transcribing the bench experiment to instructions or methods that the robot performs. This includes defining the starting setup including liquids and lab materials involved and then adding each command for the robot to perform.
Robot Setup
Defining what piece of labware goes where on the physical robot.
Run Experiment
This is a quick run-through of the experiment before start. It mainly helps visualize the flow of the experiment as well as helps debug logical or calculation errors
What mattered most to the user?
The main pain point for users was simply the lack of intuitiveness of existing software.
Reduced barrier to entry

An easier way to learn to interface with liquid handlers
Easier experiment creation

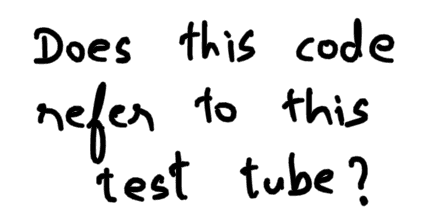

Need a more intuitive way to reference lab equipment when creating experiments
Better transparency during runtime


Need better traceability with movement of liquids, labware and reagants
Make the process of creating an experiment on the software match the mental model of the bench scientist
Lower the mental load on the user and improve error handling by making the process more visual
There were multiple approaches to improve the overall experience of designing and performing high-throughput experiments.
How would we define success?
I worked with the design lead, product manager and automation engineer in order to come up with some overarching goals and guiding objectives for this project.
Final designs

Add Materials for Experiment
The scientist inputs all the liquids and labware necessary for the experiment.
Problems targeted
Reduced barrier to entry
Easier experiment creation
Create Library of Materials
The starting contents of liquids and labware are defined in this step. This includes the starting volumes and concentrations of each liquid in a given piece of labware.
Problems targeted
Reduced barrier to entry
Easier experiment creation


Build Experiment Step-by-step
The scientist builds each step of their experiment including the source and destination, and various advanced parameters.
Problems targeted
Reduced barrier to entry
Easier experiment creation
Notes
This step is important to ensure consistency in the scientist’s mental model from their bench science to the creation of the experiment.
This feature allows the scientist to capture their thoughts at each step or upload pictures of their actual notes.
Problems targeted
Reduced barrier to entry


Experiment Simulation
A pre-run simulation of the experiment, this screen shows the location, volume and concentration of each liquid at each step of the experiment.
Problems targeted
Better transparency during runtime
Liquid Filtering
This view mode takes the point of view of the liquid. The scientist can view all the locations in which the selected liquid is present as well as its movement, volume and concentration over time.
Problems targeted
Better transparency during runtime

Challenges I faced
My internship experience was a great one. Being my first ever professional experience in UX, I learned a lot about myself as a designer, collaborator and problem solver. The internship gave me a lot of confidence in my abilities to quickly learn complex products and industries, and actually create impactful work that customers think positively about.
The internship experience did not come without challenges though. The biggest ones were:
A highly closeted industry
Getting onboarded into the industry was difficult as there were not many publicly available resources and most major players in life sciences kept their products and services close to the vest. Most companies were B2B.
Limited access to target users
I was working remotely for a smaller startup with limited customers. Therefore, my access to users was limited to those in academia and in my city.
Difficulty in measuring impact
I was working on the next version of the product while the rest of my team worked on the MVP. This made it harder to visualize and measure impact. My work has not been implemented yet.